Organizational readiness for Artificial Intelligence
- Gergely Szertics
- Jul 4, 2023
- 5 min read
Updated: Nov 10, 2023
Lately, AI has grown in popularity, largely due to OpenAI’s ChatGPT’s role in making it more accessible and practical for a wide range of people. It is a truly transformational technology that is going to change the way we think about the internet. The recent headlines, however, are just the tip of the iceberg when it comes to how AI is transforming organizations and how they should adapt. This new hype has put a huge amount of extra pressure on leaders to understand what this technology means and how they should orchestrate their organizations’ journey of living with the potentials of AI. We realized that organizations who want to successfully progress will need a well-structured road map for this journey.
The pressing questions
We have been teaching "AI in business" at Frankfurt School of Finance and Management since 2018 in Executive MBA and post experience masters and witnessed the transformation of attitude and adaptation first hand. Year by year there are more hands in the air when we ask "who has AI projects in their organization?" Year by year there is more practical interest in how specifically students can implement a certain branch of AI in their companies. We witness more awe and goosebumps around the practical use-cases and bravery around sharing first-hand experiences. More inspiring discussions are the case-studies of forerunners of adaptation trying hard to adapt these best practices to their own organizations.
And… more questions like this: “I get it, that this is how the Googles and Amazons are doing it… and it is very inspiring… But we are middle sized German company. We won’t have access to this amount of data or talent or research budget. What is OUR journey ahead?”

This question resonated well with our own experiences as consultants. Different organizational maturity stages manifest in different problems and different opportunities. Here are a few dilemmas that might be familiar:
There are too many options, how should I get started?
We have a lot of AI tools, but our people don’t use them
We have a lot of data, but we don’t know what we could use it for
We have a lot of proof of concepts, but they are not transforming into ROI
How can we comply with the data and AI regulations?
We have a lot of data, but it is very bad quality
We hired and trained AI specialists, but we can’t keep them
We have successfully implemented AI, but it remained isolated in a business unit, did not spread across other departments
We have an AI competence center, but they are detached from the business
We do implementations, but the time we get there it is outdated! How can we keep up with the pace of development?
How can we govern AI application development efforts to be ethical?
We developed AI related products, but we are not disrupting our industry, yet we could!
What the literature is saying
It is clear, that these questions are coming from companies of a different maturity levels. But how can we identify where a company is on its journey of making the most of AI? What dimensions should we pay attention to progress on this journey?
First, we turned to academic and consultancy researches where we found lots of models to describe AI maturity. Here are our top picks:
The most widely used is Gartner’s 5 stages: Awareness, Active, Operational, Systemic, Transformational. There was a great executive survey by LXT using the same dimensions that shows that 60%+ of US companies are in the first two stages.
Deloitte also created an extensive research putting organizations into a good old 2 by 2 with the dimensions: number of AI projects fully deployed and number of outcomes achieved. The 4 quadrants are Starters, Pathseekers, Underachievers, Transformers. They use this to identify success factors of achievers, that could be summarized as Invest in culture and leadership, transform operations, orchestrate tech and talent, select use-cases that can help accelerate value.
In the academic literature Schuster, Vaiderich and Volz give a great academic literature review and synthesis of scientific models resulting in 7 dimensions (Culture/mindset, Data, Ethics, Organization, Privacy, Strategy, Technology) and 5 maturity stages (Novice, Explorer, User, Translator, Pioneer)
The Swedish RISE and Gradient Descent authors created a very visual model putting Ethics in the center and adding Strategy, Ecosystems, Mindsets, Organization, Technologies, Data and define their 5 stages: Foundational, Experimenting, Operational, Inquiring and Integrated
Introducing our Organizational AI Maturity Modell
Although these are great models and tremendous insights, we found that we can’t answer the practical questions sampled above with them. Through discussions we found that both the difficulties of the stages after experimentation and the depth of dimensions are not expressive enough.
Therefore, we aggregated these insights with our own experience and elaborated a more in detailed model that could be used as a reference for understanding where companies are and direct their focus to the domains they have to improve in.
We described the winding road of maturing in AI into 6 stages with typical alternative paths and more in-depth integration of the characteristics of tech-ready organizations:
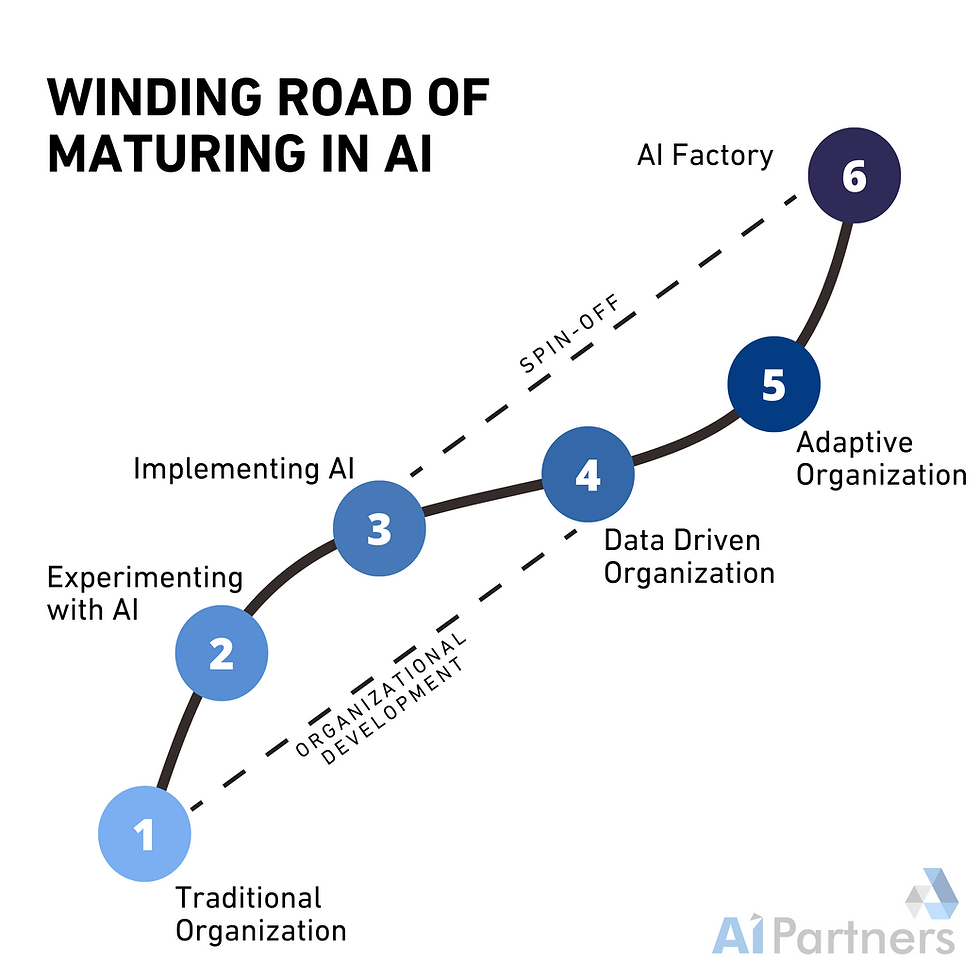
Here we give a brief description of the stages, but we will have a more in-depth about all of them:
Traditional organization: here AI is not on the radar. We don’t think there are organizations who don’t use AI (everyone is googling at least), but they are not aware of using AI, or not using it because it is AI, but it happens to be powered by AI.
Experimenting with AI: here AI is not on the strategic radar, but happens. A lot of excited people are experimenting with specific tools, with small budgets for Proof of Concepts that should prove their ROI.
Implementing AI: here AI is seen as a special purpose technology that can be implemented in a specific domain. For example implementing to customer service or marketing, or manufacturing. The key difference is that the company is willing to change its operations to use AI not just expect it to yield results.
Data driven organization: here AI is seen as a general purpose technology, that needs an organization-wide response. Using AI becomes an organizational capability with specialized skills and processes, rather than a technology that has capabilities.
Adaptive organization: here AI is understood as a technology that evolves faster than we can implement and we need processes to keep up with the pace not only getting out the most of what we have today. This shifts the organization towards an active role in the ecosystem.
AI factory: here AI is seen as an opportunity for business model innovation. The capability of providing services that are only feasible because there is AI behind it and bringing disruption to even our own industry.
Of course not all companies aim to get to level 6. In fact the working way is to aim 1-2 stages up the scale because of the inertia of the organization.
We also saw, that organizations need specifications in how they should approach their organizational AI maturity. This is why we elaborated the following 8 dimensions that are organized into technological capabilities and organizational capabilities.
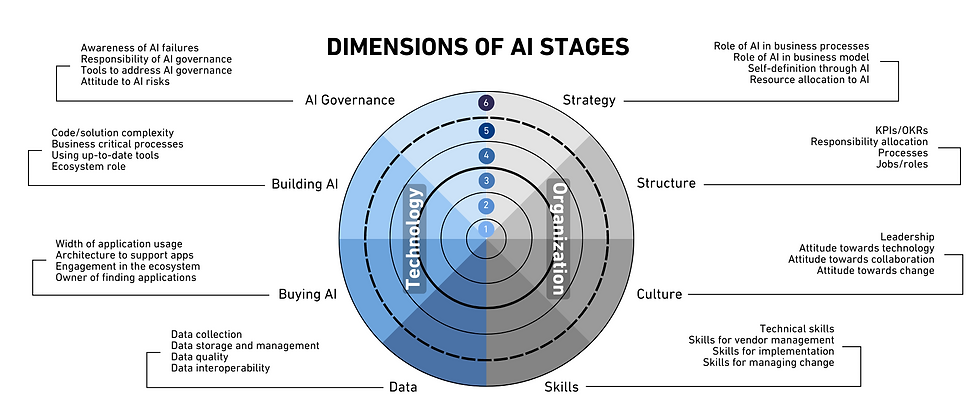
We saw that these are the dimensions in which organizational AI capability developments happen. These are where organizations differ on different maturity levels. And often times high attention to some dimensions and low on others hinder the overall effort to progress.
This is a lot of dimensions. Especially with the 4 sub dimensions each. But we wanted to create something for practitioners like ourselves, that can be explained on a higher level as well.
In the following blog posts we will go deeper into the stages and the dimensions to share insights and trigger discussions about how AI implementation is happening.
Comments